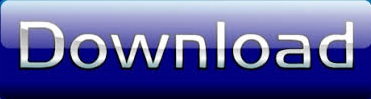
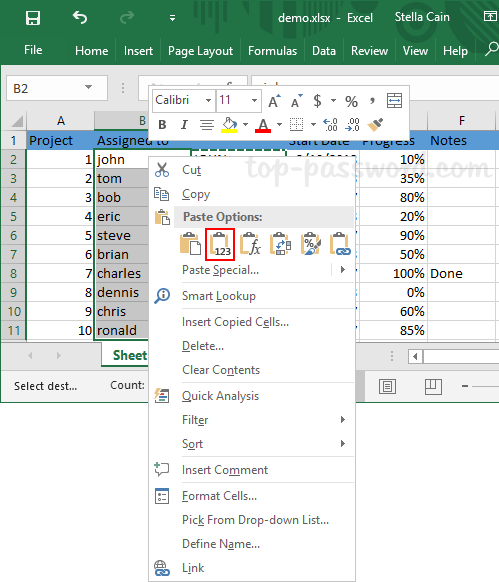
On the other hand, supervised method such as partial least squares data analysis (PLS-DA) and support vector machine (SVM) are widely used in discovering biomarkers, classification, and prediction. Examples of the analysis of unsupervised method are the principal component analysis (PCA) and clustering. This method is commonly used in preliminary evaluation of the information contained in the data sets. Unsupervised method generates data clusters without any prior knowledge about the group structure ( Ren et al., 2015). A number of available tools for metabolomics data mining are dedicated for pattern or attributes recognition and dimension reduction based on two strategies of exploration and confirmation the unsupervised and supervised methods respectively. Post-hoc analysis of high-throughput multi-dimensional data generated from metabolomics platforms may require the involvement of multidisciplinary skilled personnel, e.g., analyst, bioinformatician or scientist who are proficient in chemometric analysis.

:max_bytes(150000):strip_icc()/012-how-to-create-a-report-in-excel-889c9bba712140278816b0ec1668efdd.jpg)
The resulting metabolome profiles provide functional signatures that can be analysed using chemometrics which involves simultaneous measurement of two or more variables in an experiment to capture relationship among the variables caused by changes of metabolite abundance ( Saccenti et al., 2014). Analysis of intricate biological samples are being routinely carried out using LC-MS compared to other metabolomics approaches due to a combination of sensitivity and the amount of information generated by the analysis for instance retention time ( t R) information, mass-to-charge ratios ( m/z), signal intensities and ion abundance, which can be further used as an additional information for indexing metabolites ( Dunn et al., 2013).
#DATA ANALYSIS EXCEL MAC 2012 SOFTWARE#
Metabolome analysis is particularly employed to monitor, measure and assess the myriad diversity of various physical properties of metabolites and demands high end analytical instrumentations and software solutions, i.e., gas chromatography-mass spectrometry (GC-MS), liquid chromatography-mass spectrometry (LC-MS), capillary electrophoresis–mass spectrometry (CE-MS) and nuclear magnetic resonance (NMR) spectroscopy ( Johnson and Gonzalez, 2012). In the targeted approach, a relatively small number of metabolites of interest is to be predefined prior to running the experiment for detection and quantification. It involves profiling of metabolites with maximum metabolome coverage over a wide range of complex phytochemical structures to provide more opportunity to identify changes without bias and a priori knowledge regarding the examined specimens. The nontargeted methodologies is the most suitable technique to detect unexpected changes in phytochemical concentrations. A metabolome can be examined by two distinct approaches nontargeted and targeted analysis of endogenous and exogenous metabolites ( Brown et al., 2009). The size and complexity of plant metabolites collections, i.e., metabolome vary by species and samples ( Beisken et al., 2015). Extensive phytochemical analysis in relation to functional genomics and systems biology within an organism, tissue or cell under a given set of conditions at specific time is dubbed as metabolomics ( Sindelar and Patti, 2020). Phytochemicals are low molecular weight small molecules found in plant cells and tissues and are easily absorbed or damaged by spontaneous reactions, enzymatic reactions or conjoined with other molecules ( Zhou et al., 2012). This comparative analysis will certainly provide scientists with salient notes on data assessment and data mining that will later allow the depiction of the overall oil palm status in-situ and ex-situ. As different software tool encompasses its own advantages and limitations, the insights gained from this assessment were documented to enlighten several aspects of functions and suitability for the adaptation of the tools into the oil palm phytochemistry pipeline. In this investigation, several statistical software platforms ranging from exploratory and confirmatory technique of multivariate data analysis from four different statistical tools of COVAIN, SIMCA-P+, MetaboAnalyst and RIKEN Excel Macro were appraised using an oil palm phytochemical data set. Apposite data mining to interpret the mounds of phytochemical information from such a dynamic system is thus incumbent. Apart from genotypic traits, slight changes either by biotic or abiotic stimuli will have significant impact on the metabolite abundances and will eventually be observed through physicochemical characteristics. Recent advances in phytochemical analysis have allowed the accumulation of data for crop researchers due to its capacity to footprint and distinguish metabolites that are present within an organisms, tissues or cells.
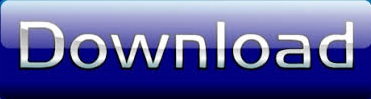